(i)
Wavelet-based structural and random noise modelling |
One substantive argument favoring the use of wavelet methods
specifically in analysis of brain imaging data that is based on the
expectation that the brain may often demonstrate broadly fractal
properties. The word fractal was originally coined by Mandelbrot (1977)
to define a class of objects with the characteristic property of
self-similarity (or self-affinity), meaning that the statistics
describing the structure in time or space of a fractal process remain
the same as the process is measured over a range of different scales.
In other words, the structure of the process is approximately scale
invariant or scale free. Many ways in which wavelets are technically
attractive for statistical analysis of fractal processes are as
follows: wavelet-based methods effect a multiresolution decomposition
that is advantageous for analysis of scale-invariant processes; (ii)
wavelet-based methods are theoretically optimal whitening and provide
KL expansions for long-memory (1/f-like) processes and many issues in
estimation and hypothesis testing are simplified by independence; and
wavelets can be used to construct good estimators for th noise process
parameters. Our contributions were on these aspects in fMRI data
analysis.
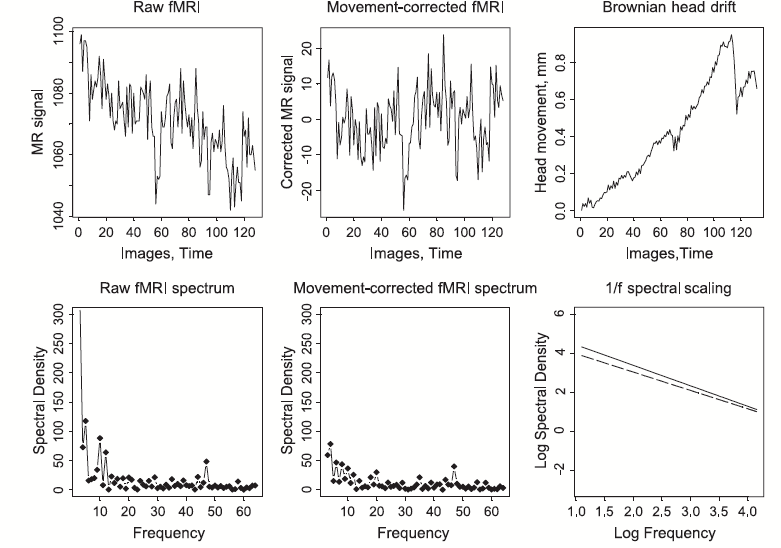 |
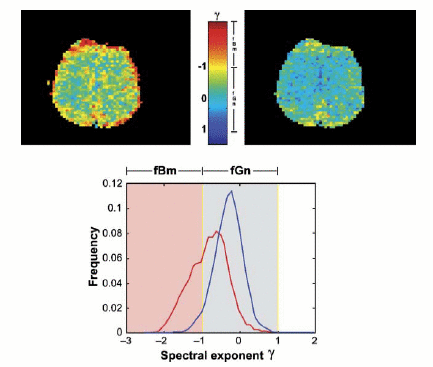 |
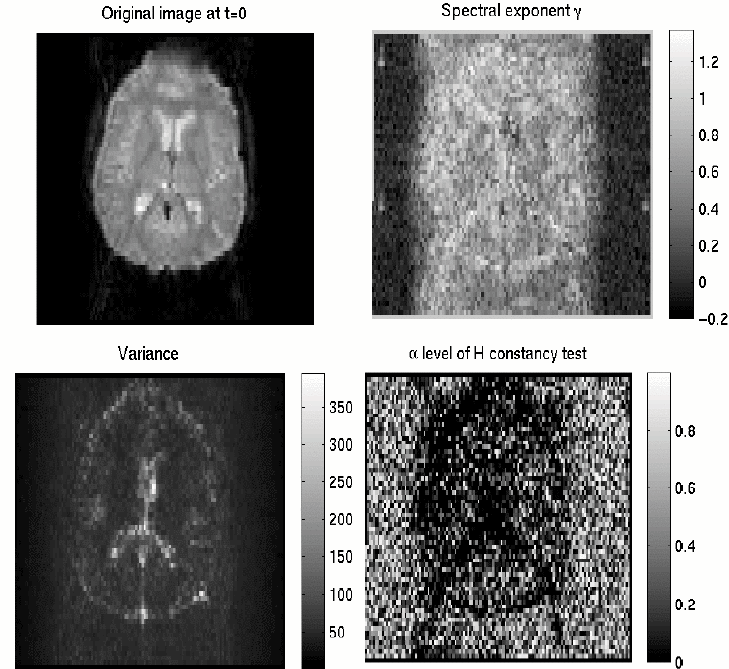 |
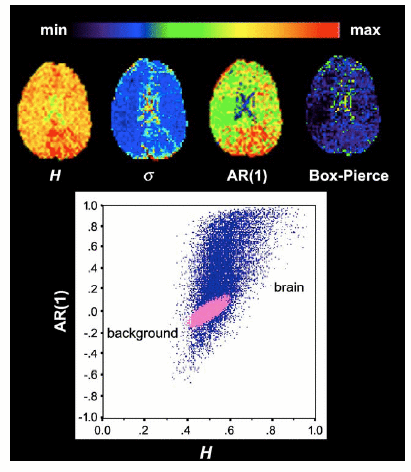 |
1/f-like
characteristics of fMRI time series. It is clear that both time series
have disproportionate power at low frequencies confirmed by the PSD
plot.
|
Spectral
exponents of fMRI data acquired under no-task conditions.
|
fMRI
data can even exhibit locally stationary 1/f behavior especially in the
cortex.
|
Note
that voxels with large Hurst exponents (or AR(1) coefficients) tend to
be concentrated symmetrically in cortical regions, whereas voxels with
small negative values tend to be concentrated around ventricles and are
associated with high variance. The swarm of (pink) points corresponding
to estimates of H and AR(1) in voxels located outside the brain, which
show that the background instrumental noise in fMRI is approximately
white.
|
|
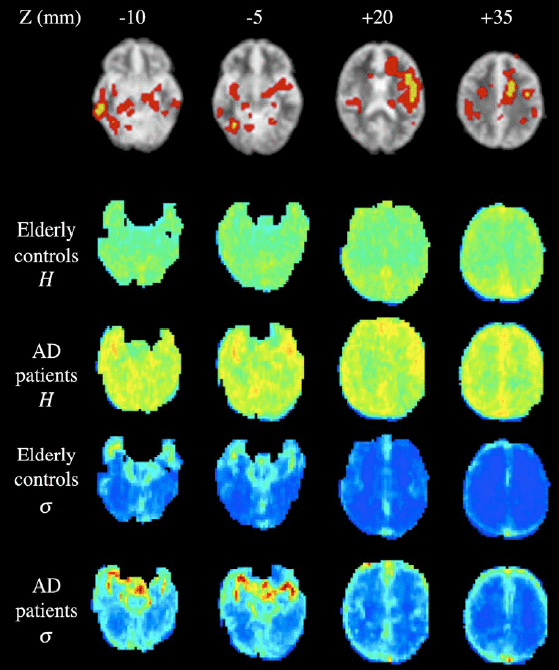 |
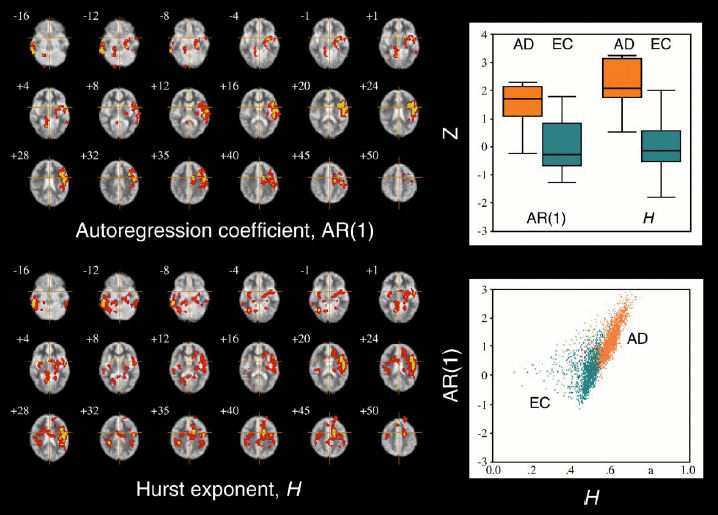 |
|
|
Effects
of Alzheimer’s disease (AD) on Hurst exponent estimated by wavelet-ML
in resting state fMRI data.
|
Whole
brain maps showing location of significant differences between patients
with Alzheimer’s disease (AD) and an elderly control group of
agematched volunteers (EC) in terms of the Hurst exponent H and the
first-order autoregression coefficient AR(1). Note the superior
sensitivity of the Hurst exponent in detecting AD-related anatomical
changes.
|
|
(ii)
Wavestrapping of 1-D time series, 2-
to 3-D spatial maps and 4-D spatiotemporal processes |
Data resampling by permutation or bootstrap offers many advantages
for inference on functional neuroimaging data—in particular, it
obviates the need to make unrealistic assumptions about spatial
auto-covariance and other distributional aspects of the data. Perhaps
for these reasons, an appropriate nonparametric test can have superior
sensitivity compared to a parametric alternative. Moreover, there are
many statistics of potential interest in brain mapping, for example,
spatial and multivariate statistics, that do not have theoretically
tractable or well-established distributions under the null hypothesis
and therefore cannot properly be tested parametrically. In contrast,
almost any statistic of interest may be accessible to inference on the
basis of an appropriate resampling scheme (for examples, respectively,
of resampling spatial and multivariate statistics in brain mapping.
However, designing an appropriate resampling scheme for statistics
estimated by analysis of a functional MRI time series is complicated by
nonindependence of the observations under the null hypothesis. Owinf ot
its decorrelating (and even stationarising) properties for a wide class
of processes, orthogonal wavelet-based transforms provide an
alternative device for the strategy of resampling. We have demonstrated
the theoretical and practical advanatges of wavestrapping in
a series of publications, for a variety of processes including 1/f and
seasonal processes.
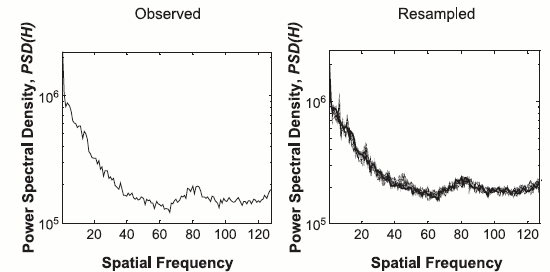
|
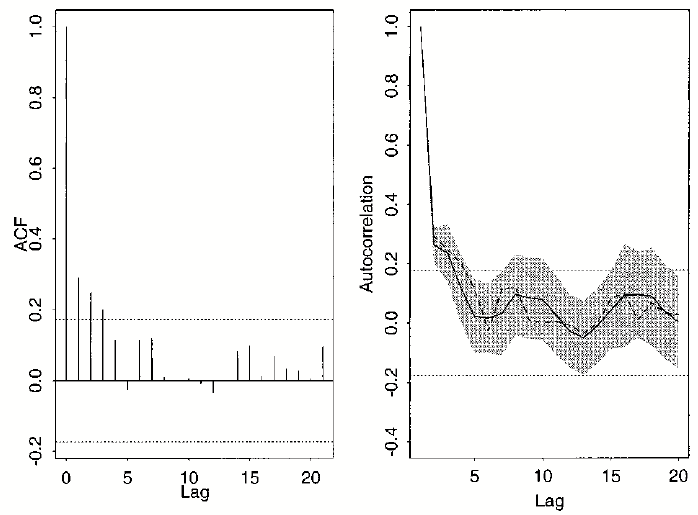
|
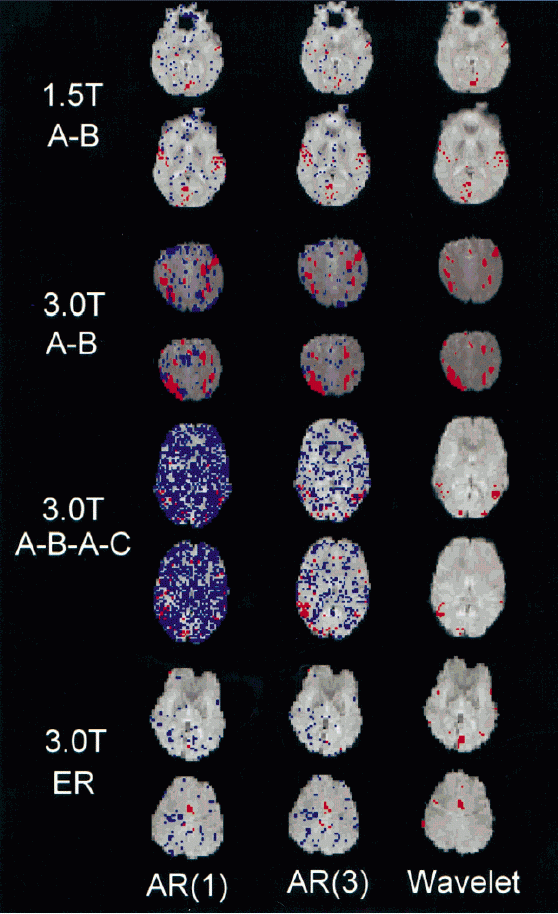
|
Wavelet
resampling in the original (spatial, temporal) domain preserves the ACF
and the PSD of the stationary original data.
|
Brain
activation mapping of experimental fMRI data based on
different treatments of residual autocorrelation. Wavelet resampling is
considerably more robust than the AR prewhitening
schemes in dealing with some of the higher field datasets,
but of approximately equivalent sensitivity.
|
(iii)
Wavelet-based estimators for parametric and semiparametric models |
We have specified the fMRI regression problem with long-memory noise
and possibly with a structural non-parametric component directly in the
wavelet domain. As noted earlier, the orthonormal DWT is approximately
a Karhunen–Loève expansion, which allows to whiten the noise.
Simuiltaneously, the sparsity of the wavelet transform ensures a
parcimonious representation of the nonparametric unknown part.
Efficient algorithms for estimating both the noise and the signal
(parametric and non-parametric parts) were proposed and theoretically
studied.
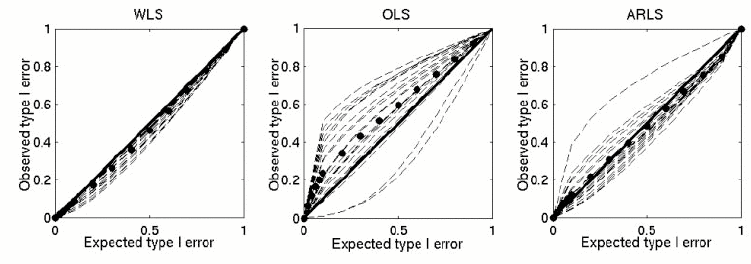
|
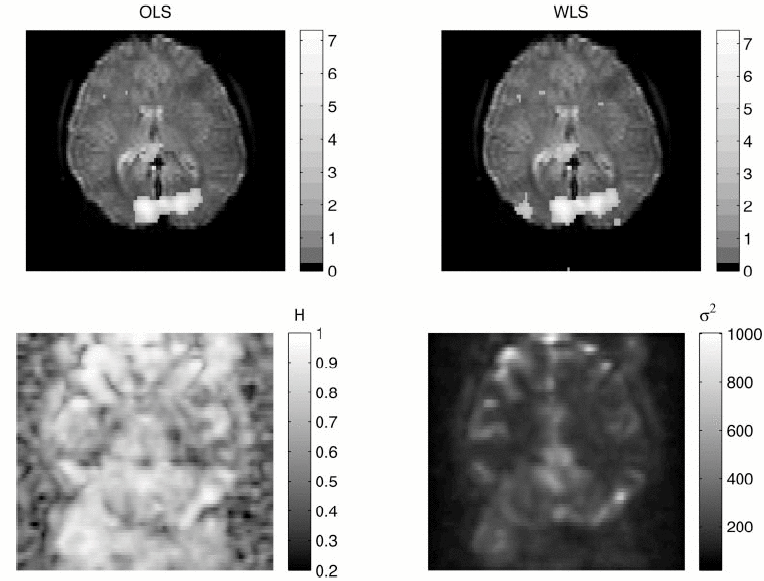
|
|
Type
I error calibration curves. Note the superiority of the wavelet-domain
LS approach.
|
Signal
and noise parameters maps for a visual task.
|
Semiparametric
models (coming soon).
|
(iv)
Wavelet-domain hypothesis testing
for estimation of spatially extended
statistic map |
We proposed a framework combining wavelet-based methods and statistical
hypothesis testing for activation mapping of human fMRI data. In this
approach, we emphasise convergence between methods of wavelet
thresholding or shrinkage and the problem of hypothesis testing in both
classical and Bayesian contexts. Specifically, our interest was focused
on the trade-off between type I probability error control and power
dissipation. We presented a technique for controlling the false
discovery rate at an arbitrary level of error in testing multiple
wavelet coefficients generated by a 2D discrete wavelet transform (DWT)
of spatial maps of fMRI time series statistics. We also described and
applied change-point detection with recursive hypothesis testing
methods that can be used to define a threshold unique to each level and
orientation of the 2D-DWT, and Bayesian methods, incorporating a formal
model for the anticipated sparseness of wavelet coefficients
representing the signal or true image. The sensitivity and type I error
control of these algorithms were comparatively evaluated by analysis of
null images and an experimental data set acquired from normal
volunteers during an event-related finger movement task. We showed that
all three wavelet-based algorithms have good type I error control (the
FDR method being most conservative) and generate plausible brain
activation maps (the Bayesian method being most powerful). We also
generalised the formal connection between wavelet-based methods for
simultaneous multiresolution denoising/hypothesis testing and methods
based on monoresolution Gaussian smoothing followed by statistical
testing of brain activation maps.
Click on each link to download
the corresponding movie (activation volume rendering).
FDR-based
map.
|
Recursive
testing map.
|
Bayesian
testing map.
|
SPMM
FWHM=6mm |
SPM
FWHM=10mm |
All maps
were thresholded at a significance level of 0.01 (height-corrected for
SPM). Note that significance of the ipsilateral cerebellar signal is
conditional on kernel size and that plausible signals in medial
premotor cortex and ipsilateral somatosensorimotor cortex recovered by
multiresolution analysis/ multiple hypothesis testing in the wavelet
domain are not evident in these results of monoresolution smoothing
followed by multiple hypothesis testing in the spatial domain.
|